Using Machine Learning to Promote Fairness and Efficiency in Indian Courts
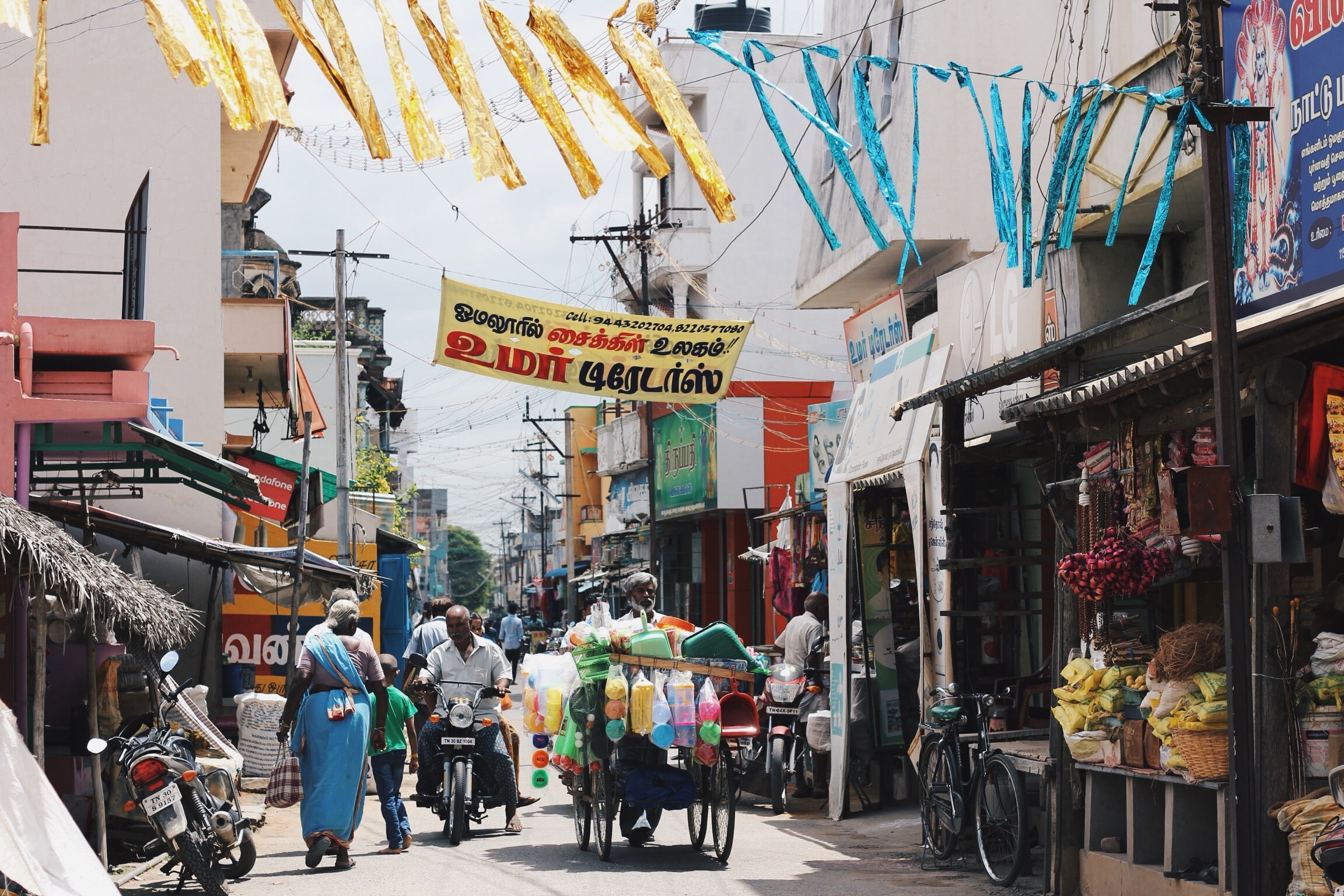
Biased or slow rulings from judges have serious economic and welfare consequences, including a harmful environment for business. Courts in developing countries face numerous challenges in providing fair and efficient justice to citizens and firms. The challenges include: legal codes burdened with formalism and out of touch with local languages, judges who are biased against plaintiffs from certain groups, preferences for informal institutions, and low human capital in the court system. This project provides empirical evidence on the extent of discrimination and delay in Indian courts, focusing on unequal treatment due to corruption and due to prejudice against disadvantaged groups (based on gender, religion, and caste).
Questions:
- Can machine learning be applied to troves of administrative court data to find instances of injustice and biased rulings by judges?
- Do judges discriminate against marginalized groups and/or favor politicians in their rulings?
Abstract:
The researchers are constructing a new dataset on the universe of judicial proceedings in Indian courts. This dataset, the first of its kind in a developing country, includes digitized records of roughly 80 million cases over a 110-year period. Adapting machine learning methods, researchers will predict judicial outcomes based on case characteristics (such as the type of felony, the plaintiff’s legal history, etc), and then identify cases with divergent rulings, labelling them as potential sources of errors or bias. The researchers will also analyze implicit biases expressed in the language text written by judges. The researchers can then look at whether, holding case characteristics constant, judges (1) discriminate against women, Muslims, and members of certain castes, and (2) favor the politically advantaged (criminally-accused politicians) in their rulings.
This team is doing a related project in Kenya. To continue making progress during the global pandemic (which suspended Kenya’s court functioning), the team has shifted their approach to focusing on the text-analytics of court data and non-experimental analysis of case-level data of hundreds of thousands of judicial cases in Kenya to identify the presence of gender bias in judicial rulings.